What is a Logic?
In the context of Doofinder's Recommendations, a Logic refers to the specific set of rules and configurations that determine which products are shown in the carousels.
Each Logic responds to different scenarios or business objectives, such as displaying products based on popularity, similarity, user behavior, or personalized by the business goals, among others. Therefore, a Logic is a tailored approach to how the recommendation engine processes data to deliver relevant products to users, enhancing the personalization of the discovery experience.
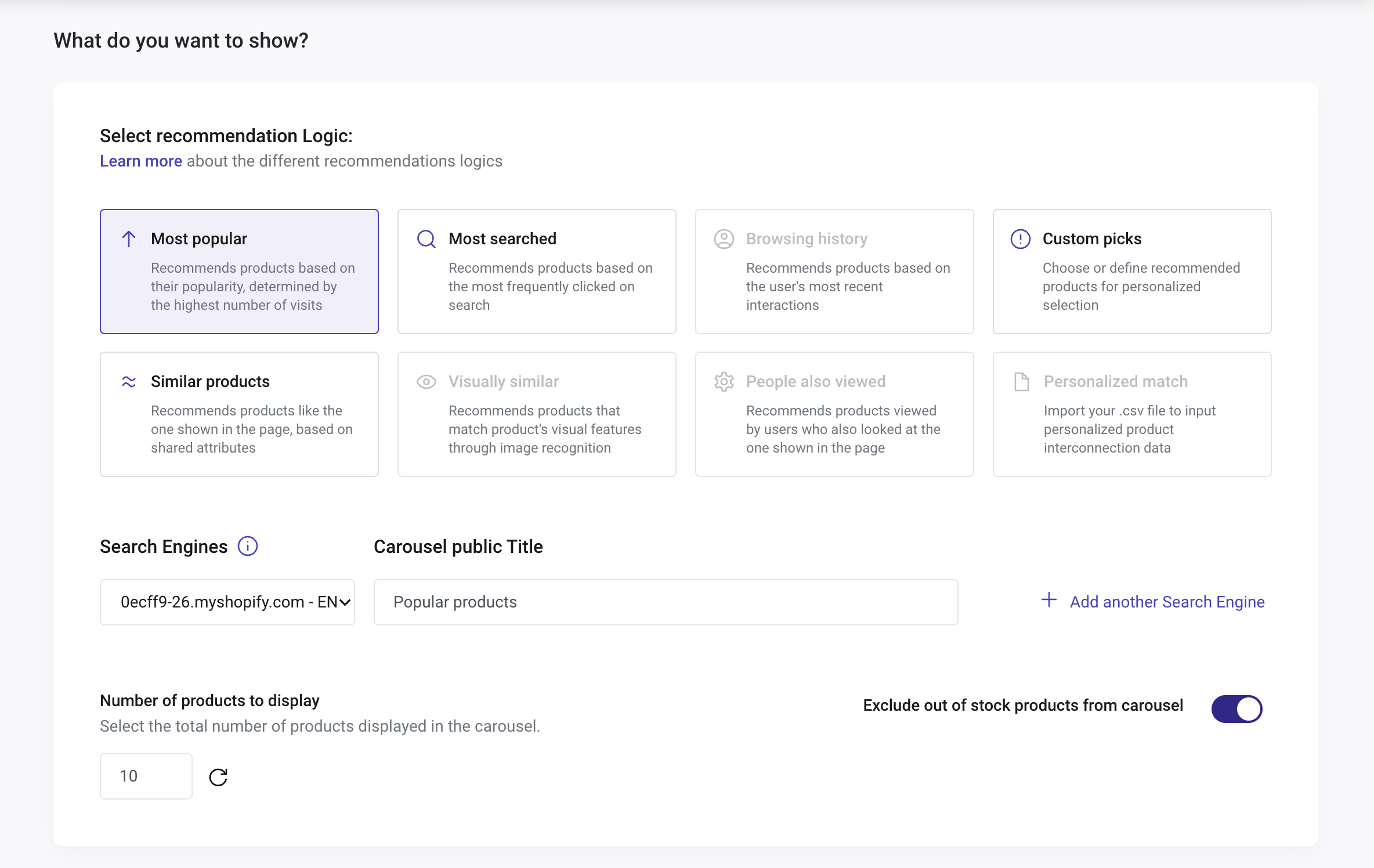
Take a look at this chart for a quick reference on each logic. Keep reading to know each logic in depth.
Quick Reference Chart Carousel Logics
Carousel Logics | Type | Recommends | Update |
---|---|---|---|
Most Popular | All Pages | Top-clicked on the whole website in the latest 15 days | Cached once per day |
Most Searched | All Pages | Most frequently clicked on the Layer in the last 15 days | Cached once per day |
Browsing History | All Pages | User’s most recent interactions | Per session |
Custom Picks | All Pages | Personalized selection: include or exclude products to show in the carousel | Depends on your rules |
Similar Products | Product Page | Based on shared attributes (title, description, brand, category) | Depends on the configuration of attributes of the feed |
Visually Similar | Product Page | Matches the product’s visual features through image recognition | Real time |
People Also Viewed | Product Page | Products viewed by users who also looked at the one shown on the page | Every 7 days by training |
Personalized Match | Product Page | CSV file to input personalized product interconnection data. Products related to each other in your eCommerce | Training. Displays when ready |
These Logics follow different rules to work. Some of them need time to train in order to display the correct products; some of them display in all Pages, and others are only available for pages that show one single product.
In the following sections, we break down each of them for a proper use and understanding.
If you already know which Logic to use and want to take a look at its characteristics, go straight to them below:
If you want to apply a different logic to the carousel you have already created, remember that once saved, these cannot be modified. To make changes, you will need to create a new carousel and apply the desired logic.
Logics for all Pages
Most Popular
Recommends products based on top clicked on the whole website in the latest 15 days. This logic updates every 15 days.
Most Searched
Recommends products based on the most frequently clicked-on search layer in the last 15 days.
Browsing History
Recommends products based on the user’s most recent interactions. To display needs 1 product to be seen by the user.
This logic shows products according to the user session. Learn more on sessions.
Custom Picks
Choose or define recommended products for personalized selection. Include or exclude products to show in the carousel through rules or by adding individual items.
Logics for Pages that show one single product
All the logics that only work for single product pages cannot be selected in the case of choosing a Home page, Category page, or Shopping Cart page. However, they can be selected when choosing a Specific page or a Custom page. But, if the page you add as Custom or Specific is not a Product page, then the widget won’t work.
Similar Products
Recommends products similar to the one shown on the page based on shared attributes (title, description, brand, category).
Visually Similar
Recommends products that match the product’s visual features through image recognition. The products shown are updated on real time with a similar product to the one viewed.
Note that for this logic Doofinder only considers the exact image. As a result, if a product has multiple variants, the logic will prioritize the variant that visually matches the input the most closely.
Suppose your catalog lists a white t-shirt as the parent product with variants in red, blue, and green. If a user searches for another kind of red shirt, the Visually Similar logic will evaluate the red t-shirt variant rather than the white t-shirt or any other color. This ensures that results are aligned with the specific visual input.
People Also Viewed
Recommends products viewed by users who also looked at the one shown on the page. This logic needs training to function properly. Read in this section how it trains.
Personalized Match
Import your .csv file to input personalized product interconnection data. In this case, upload a .csv file that indicates which products in the eCommerce store are related to each other (one .csv for each Search Engine). The system uses this data to train its logic, enabling the carousel to display products related to the one currently shown on the page. To learn more about the training process of Personalized Match, read this section.
Requisites for utilizing a Personalized Match:
This logic requires a large amount of data to be stored in a .CSV file. If you are working with a database or spreadsheet, export your .CSV file with the list of URLs.
List of URLs specification: in your .CSV file, a list of URLs must be displayed and separated by spaces. It should look like this:
url1 url2 url3 url4
url5 url6 url7 url8 url9
These URLs should match articles that are indexed in your feed. This will allow Doofinder to identify and group related URLs from a client's feed, ensuring they are valid product URLs, and disregard any irrelevant ones. For this logic to work properly make sure to have at least 401 rows. Each product needs at least 4 rows.
Logics with Training
Two of the Recommendations Logics require learning with a training period in order to work and display. The first one is Personalized Match, and the second one is People Also Viewed.
The training phases are Learning, Ready or Failed. The carousel will show products only once the Logic is ready. Learning for these logics behave differently because they gather information from distinct sources. The training process is also diverse between both of them:
Personalized Match
Personalized Match retrieves the information from the data feed of your .CSV file. This logic will train in order to display correctly.
Three different states apply to the training process of Personalized Match:
-
Learning: After your .CSV file is uploaded, training begins. This status will show as long as it needs to before completion. Once the training is finished, two other status can appear: Ready or Failed.
-
Ready: The training worked properly, and now Personalized Match is working on your Recommendations Carousel.
-
Failed: If the training doesn’t work, this status will appear. Failed means that you don’t have enough data in your .CSV file, or it doesn’t have enough quality data to work properly. Upload another .CSV file for another training.
People Also Viewed
People Also Viewed retrieves the information from the data generated from your user’s behavior. This logic will train in order to display correctly.
Two different states apply to People Also Viewed:
-
Learning: this status will appear once this logic is selected. The learning period collects data every seven days. If unable to collect enough data it will train again on the date displayed.
-
Ready: Once the learning is completed it will show as ready and display correctly on your website. People Also Viewed will train every seven days to be updated with the last data generated.
To ensure your carousel displays products even while these logics are training, enable Fallback for the Most Popular logic to populate the carousel. If Fallback is disabled while these logics are training, the carousel will not appear.
Training specifications
People also viewed, and Personalized match use a model called Word2Vec with a training threshold to show the desired results.
What is Word2Vec?
Word2Vec is a method developed to transform words into numerical vectors so that words with similar meanings have similar vector representations. This allows machines to understand the context and relationships between words more effectively.
For Recommendation, a similar approach can be used where instead of words, we consider user sessions and the products viewed within those sessions. This applies to our Recommendation Carousel as Word2Vec can be adapted to recommend products based on the similarity of viewing or purchasing patterns.
How Does the Model Threshold Work?
Word2vec training:
The model trains based on user sessions.
A session is a set of pages that a user visits during a period of time. Nowadays, that period is 12 hours. These sessions are used as training data for the Word2Vec model to learn the relationships between products.
Training Requirements
-
Sessions: A session is a set of user interactions within 12 hours.
-
Threshold Ratio:
- Formula: Sessions ÷ Unique products seen
- Requirement: A ratio of at least 4.0 (there must be 4 times more sessions than different products seen).
- Reason: Ensures enough data diversity for accurate training and reliable recommendations.
Having a higher ratio means the model sees each product in various contexts, improving its understanding of relationships between products, leading to better recommendations.
More sessions provide diverse user interactions, enhancing the model's training quality. A ratio above the threshold ensures that the model has enough training data to create reliable embeddings.
Example Cases
Case 1
Bob has a store of 80,000 products.
There are 100 different sessions as there are 100 different users searching for products.
Within those sessions, 5,000 unique products are seen.
Ratio: 100 / 5000 = 0.02 (below 4.0)
Case 2
Bob has a store of 800 products.
There are 100 different sessions as there are 100 different users searching for products.
Within those sessions, 24 unique products are seeing.
Ratio: 100 / 24 = 4.167 (meets threshold).
More sessions are required when more unique products are viewed to maintain the threshold. This means, having more people view the same products will improve the ratio, whereas if more people view different products, you'll need additional visits to the same products to improve the ratio.
Personalized Match Specifications
For Personalized Match to work well, it needs good quality data, meaning useful and sufficient information. This means having a populated file of products.
To work properly, make sure that the .CSV file contains at least 4 rows + 1 per product. Therefore, if you have a catalog of a 100 products, you will have a file of a 401 rows.
People also Viewed Specifications
People also Viewed works by transforming the images into vectors using a model and indexing them in OpenSearch, right after indexing the feed. When someone searches using an image, we also transform that image into vectors and perform a KNN search, which returns the most similar images for that feed (by finding the k nearest vectors to a query vector, measured by a similarity metric). Essentially, just as we index titles, descriptions, etc., we also index the images.
First, Logic. Then, Filters.
Filters are used to select products within the selection of items powered by the logic (e.g. within the logic “Most Popular”, I can filter for the brand “Nike”).
Generate more value with your desired logic by applying Filters. Learn more in Filters and Fallback.